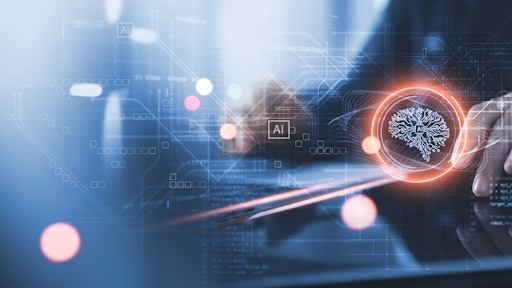
tippapatt.stock.adobe.com
As of April 2023, the U.S. Department of Transportation reported that there were over 750,000 active carriers in the U.S. that own or lease at least one tractor. Even though experts suggest we are now at the bottom of the market and demand will only increase, to say that the market for freight is fierce is an understatement. The financial reserves that fleets had built up during the Covid pandemic freight boom are now nearly depleted and trucking companies’ current bidding processes may determine if they’ll survive and thrive or need to shut their doors.
Freight bidding season refers to the time in which shippers and carriers negotiate freight rates and contracts for the upcoming season. Typically, this corresponds with peak season beginning in Q4 and increasing into Q1 but can vary depending on the industry and location. It can be a complex and competitive process that involves careful planning and analysis for both shippers and carriers. Shippers need to consider multiple factors like cost, reliability, and volume, while carriers need to assess profitability and risk.
The strategy used to decide which lanes to bid on and at what rates can be the difference between profit and loss in an industry that operates on tight margins. A study by the American Transportation Research Institute (ATRI) found that the average operating ratio for trucking companies in 2019 was 98.7%, indicating that carriers spent $0.987 on operating expenses for every $1.00 earned. Much more of an art than a science, the bidding process is often far from straightforward. It can be a lengthy, manual process that can take teams of analysts a significant amount of time to determine the right strategy and order based on the nearly unlimited number of lane combinations.
This is where artificial decision intelligence can help support trucking companies with a better informed and time-saving approach to bid analysis that accounts for current and historical market data. By funneling in and analyzing information from the market, the trucking company’s capacity, and network balance, an artificial decision intelligence platform can help make informed, optimized decisions.
Bidding and RFP Challenges
Most bidding opportunities occur in a yearly request for proposal (RFP) process in which a shipper puts out a request for a carrier or 3PL that can best fit their needs. This can either be a renegotiation with a current carrier or 3PL or for a new one. The RFP process itself brings several challenges for trucking companies, driven by the fact that many shippers are going through the same process at the same time and there are a multitude of different options. Trucking companies struggle with limited time to assess their network capabilities and capacity along with current market rates to ensure they are answering with a competitive price while remaining profitable. The high volume of bids can also result in carriers bidding on the wrong lanes for their network which can impose profit risks by positioning their assets in low-opportunity regions or market imbalance issues.
One of the most significant challenges trucking companies and asset-based 3PLs face during the bidding process is balancing cost and revenue. They also must consider the risks of certain loads and how it could potentially impact their ability to deliver the loads on time and profitably. Without the use of intelligent tools, carriers run the risk of committing to contract volume that they cannot handle. The cost of empty miles, current commitments, driver parameters, as well as the shipper’s reliability all must be factored in. Carriers also need to balance optimizing their network by maximizing the use of trucks, drivers, and trailers to minimize empty miles and increase revenue.
Bidding Process Troubleshooting
With a traditional approach to bid analysis, the difficulty lies in being unable to simulate the effects on the current network and the potential impacts (good or bad) on profit margins, network balance, and service coverage among others. To fully understand profitability, bid analysts must consider all load factors within the context of the broader network and company objectives. All of these factors have the potential to impact the bottom line significantly yet are often left out of the manual data interpretation and decision-making process.
Trucking companies that are able to translate lane-level options into load-level activity are the ones that can truly understand the effects on their network and profits. To achieve this level of insight, they need simulation capabilities as well as historical data to serve as a reference guide, translating the hypotheticals into real possible scenarios of load coverage, profits, and more. This would allow companies a window into the future to view how analyzed bids would impact their existing network and create future predictions that would accommodate the impact of incorporating the new bids.
How AI Can Help
An artificial decision intelligence-powered solution completely handles the heavy lifting of bid analysis in a way that is not possible through human interpretation. Carriers gain better clarity on the lanes that bring balance and monetary growth to their business, the impact of each lane on their existing network, and uniquely valuable lanes. These detailed insights give trucking companies a way to approach the bid analysis process faster and more efficiently, especially when trying to compare and handle multiple RFPs at a time.
The power of network simulation when it comes to bidding analysis helps carriers understand their network’s performance and forecast growth and profitability outcomes of different scenarios:
- Improved decision-making – Analysis tools provide insight into load and lane profitability so carriers can decide which bids will be most profitable based on network balance. Lanes that may not be high in value still contribute to balancing your network and positioning assets in high-priority markets.
- Efficient resource allocation – Better insight into driver availability, equipment, and routes, allowing for increased fleet utilization, reduced empty miles, and more cost-effective use of assets.
- Enhanced pricing strategies – Access to historical data and network impact simulations, allows carriers to focus pricing strategies on the lanes that set their network up for success.
- Proactive network adjustments – Continuous optimization and adjustments to account for potential risks and disruptions in the network, in order to maintain service levels and minimize the impact on the bottom line.
- Better partner collaboration – Increased communication and collaboration between supply chain partners for greater operational efficiency.
- Increased customer satisfaction – Ability to better meet their customer promises of on-time delivery rates which increases overall customer satisfaction.
- Increased driver satisfaction – Factors in driver preferences, improving home time, and increasing paid mileage allow carriers to build better relationships to improve driver retention.
In addition, artificial decision intelligence technology can also share the “why” behind each decision, giving operators more insight into the potential effects on their network. Rather than replace decision-makers, AI supplements their awareness. AI technology allows users to remain in control over any final adjustments and negotiations based on data and simulations to help them make final decisions.